Data Analytics in the Financial Market? Tools and Methods
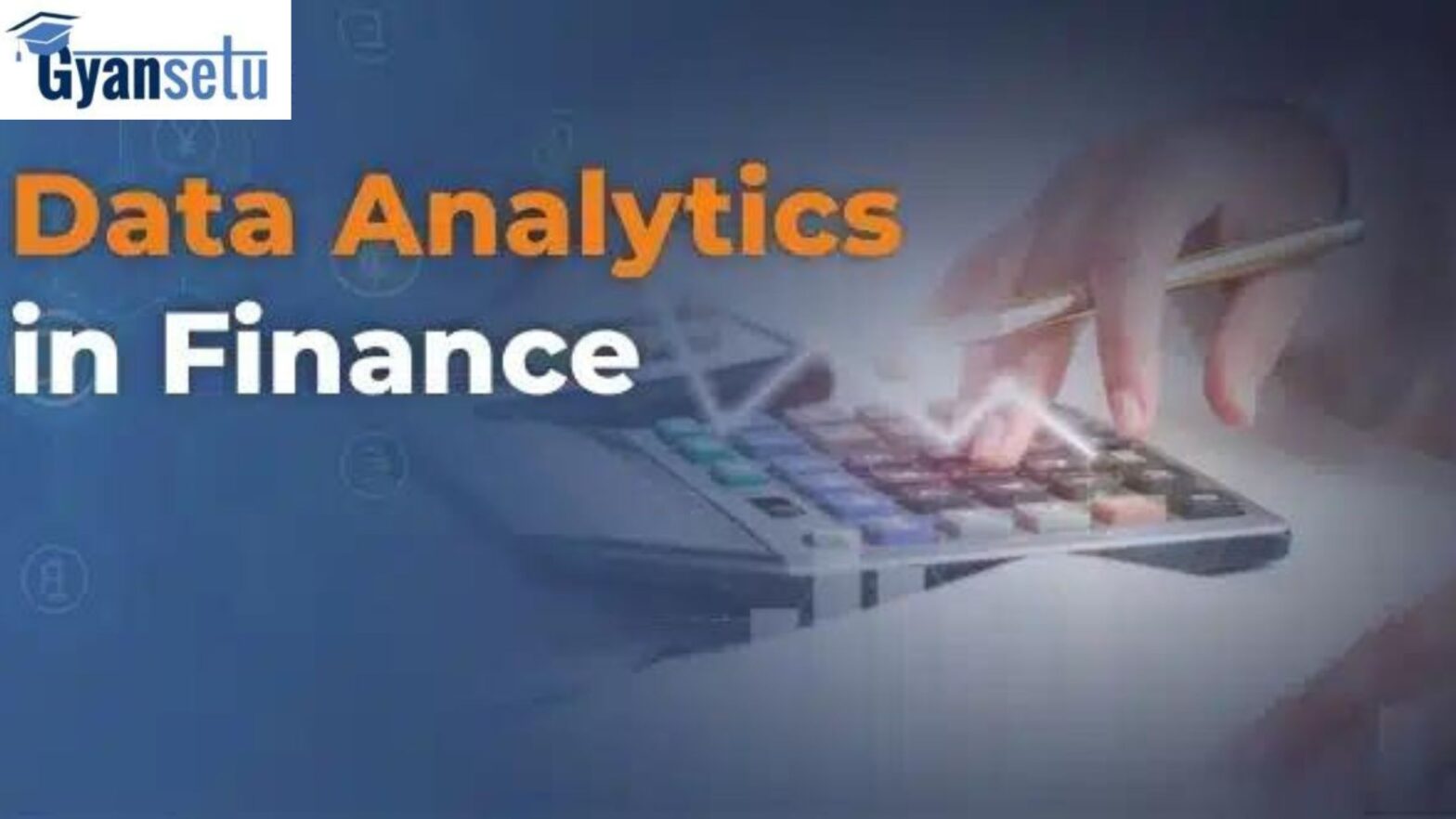
As the volume and variety of financial data continue to grow, the requirement for effective tools and methods to analyze and derive meaningful insights has become paramount. Data analytics is revolutionizing decision-making processes, risk management, and investment strategies in the financial sector.
This article delves into the tools and methods employed in data analytics within the financial market, exploring their applications and impact.
The Rise of Data Analytics in Finance
Normally, financial decision-making heavily relies on human intuition and experience. But, with the arrival of big data, the financial industry has undergone a major transformation. Data analytics, including various techniques and technologies, has emerged as a game-changer. It enables financial institutions to harness the power of data for better decision-making, risk assessment, fraud detection, and customer satisfaction.
This shift in the industry has changed the way financial services operate and improved adaptability to different market conditions. By using advanced analytics tools, institutions will be able to adapt to huge datasets and extract valuable insights they could not get previously.
Also, this analytical skill has empowered financial professionals to make more informed and strategic decisions, driving them away from reliance solely on intuition. As the financial landscape evolves, big data analytics is poised to play an increasingly pivotal role in shaping the industry’s future.
The newfound reliance on data-driven insights represents a departure from traditional methodologies, underscoring the industry’s commitment to staying ahead in an era of technological innovation.
Tools in Data Analytics for Finance
-
Business Intelligence (BI) Tools
Business Intelligence tools are fundamental in data analytics for the financial market. These tools, such as Tableau, Power BI, and QlikView, enable users to visualize and interpret complex data sets.
In finance, these tools can be utilized for creating interactive dashboards, reports, and visualizations that provide insights into market trends, portfolio performance, and risk factors. BI tools empower financial analysts to make informed decisions by presenting data in a user-friendly and understandable format.
-
Statistical Analysis System (SAS)
SAS is a powerful statistical software suite widely used in the financial sector. It offers a range of tools for data management, advanced analytics, and predictive modeling.
Financial institutions leverage SAS for credit risk analysis, fraud detection, and compliance reporting. The software’s ability to handle large datasets and perform complex statistical analyses makes it indispensable in the data analytics toolkit of financial professionals.
-
R and Python Programming
Programming languages like R and Python have gained immense popularity in the financial industry due to their versatility and extensive libraries for data analysis.
Financial analysts use these languages to build custom models, perform statistical analysis, and develop machine learning algorithms. R and Python enable the creation of predictive models for stock price forecasting, credit scoring, and other critical financial predictions.
-
Machine Learning Frameworks
Machine learning plays a pivotal role in data analytics within the financial market. Frameworks such as TensorFlow, PyTorch, and scikit-learn enable the development of sophisticated models for tasks like algorithmic trading, fraud detection, and sentiment analysis. These frameworks leverage complex algorithms to analyze historical data and make predictions, helping financial institutions stay ahead in a rapidly changing market.
Methods in Data Analytics for Finance
-
Descriptive Analytics
Descriptive analytics involves exploring historical data to understand patterns, trends, and anomalies. This method helps analysts gain insights into market behavior, customer preferences, and investment trends in finance. Financial professionals can uncover valuable information that informs strategic decision-making by utilizing techniques like data aggregation and data mining.
-
Predictive Analytics
Predictive analytics utilizes statistical algorithms and machine learning techniques to identify future trends and outcomes based on historical data. In the financial market, predictive analytics is widely used to forecast stock prices, assess credit risk, and optimize investment portfolios. Financial institutions can make informed predictions and mitigate potential risks by analyzing patterns and identifying correlations.
-
Prescriptive Analytics
Prescriptive analytics goes beyond predictive analytics by recommending actions to optimize outcomes. In the financial sector, prescriptive analytics assists in decision-making by providing insights into the potential impact of different strategies. For example, it can help financial institutions determine the optimal allocation of assets in an investment portfolio or recommend adjustments to risk management strategies based on changing market conditions.
-
Text Analytics
Text analytics involves the extraction of insights from unstructured data sources, such as news articles, social media, and financial reports. Sentiment analysis, a subset of text analytics, helps gauge market sentiment and investor perceptions. By analyzing textual data, financial institutions can stay next to market developments, assess public opinion, and make timely decisions to capitalize on emerging opportunities or mitigate risks.
Applications of Data Analytics in Finance
Must Read – Why jobs in data analytics are increasing
-
Algorithmic Trading
Algorithmic trading, also known as algo-trading, relies on algorithms to execute trades at optimal prices and speeds. Data analytics is integral to algorithmic trading, as it involves processing vast amounts of market data in real-time to identify trading opportunities and execute orders swiftly. By leveraging historical data and market indicators, algorithmic trading systems aim to capitalize on price differentials and market inefficiencies.
-
Risk Management
Risk management is a critical aspect of financial operations, and data analytics plays a major role in identifying and mitigating risks. Through advanced analytics, financial institutions can assess credit, market, and operational risks. Predictive models help anticipate potential financial downturns, enabling proactive risk mitigation strategies.
-
Fraud Detection
The financial sector is particularly susceptible to fraudulent activities, and data analytics is a potent tool in fraud detection. Machine learning models can analyze transaction patterns, detect anomalies, and identify potential instances of fraud in real time. These models enhance their ability to detect sophisticated fraudulent activities by continuously evolving and learning from new data.
-
Customer Segmentation and Personalization
Data analytics enables financial institutions to understand their customers better. Through customer segmentation, institutions can tailor their services and products to meet different segments’ specific needs and preferences. Personalization based on data analysis enhances customer satisfaction, fosters loyalty, and drives business growth.
-
Regulatory Compliance
Compliance with regulatory standards is a constant challenge for the financial industry. Data analytics assists in ensuring adherence to regulations by providing monitoring, reporting, and auditing tools. Financial institutions can navigate the complex regulatory landscape more effectively by automating compliance processes and analyzing data for potential regulatory issues.
Challenges and Future Trends
While data analytics has brought about significant advancements in the financial market, challenges persist. Data privacy concerns, the need for skilled professionals, and the constant evolution of technology are among the challenges financial institutions face in effectively leveraging data analytics.
The inclusion of artificial intelligence (AI) and blockchain technology is anticipated to shape the future of data analytics in finance. AI-powered analytics tools will become more sophisticated, providing deeper insights and automating complex decision-making processes. With its decentralized and secure nature, blockchain will enhance data integrity and transparency, addressing concerns related to data tampering and fraud.
Final Verdict
Data analytics has become a cornerstone of success in the financial market, transforming how institutions operate and make decisions. The tools and methods discussed in this article, including “Data Analytics in Finance,” empower financial professionals to harness the vast amounts of data available, turning it into actionable insights.
As technology advances, the future holds even greater possibilities for financial data analytics, promising more accurate predictions, improved risk management, and enhanced customer experiences. So, with Gyansetu, learn data analytics in finance, as it is an essential skill nowadays and offers vast opportunities in the future.
Moreover, financial institutions that embrace and master data analytics will likely be at the forefront of innovation and competitiveness in the dynamic landscape of the financial market.